AutoMetis is pleased to unveil its proprietary automated trading strategy "Athena": a systematic method to monetize fundamental technical properties of FX markets.
Athena was conceived in 2015, and had a prototype launched into live trading in the FX market later that year. It has since received two major overhauls: one in late 2017 relating to an improvement in its execution algorithm resulting in savings in transaction costs, and one in early 2020 relating to its trade order diversification mechanism and to a recalibration of its risk framework to bring it in line with the market standard.
We present below in detail the underlying concept, the backtested performace, our live track record, and the risk analysis of our stress-testing simulations.
- Absolute return strategy constructed to achieve a high-certainty return target
- Medium frequency, fully automated trading over major USD crosses
- Uncorrelated with equity market; scalable and flexible risk profile
Athena exploits the existence of fat tails in the distribution of FX price changes in the medium term — the empirically-supported proposition that large market moves occur more frequently than normally.
At the same time, the academic literature finds in the FX market the least evidence for the existence of mean reversion, further fuelling the expectation that deviations from the mean tend to be large and lasting, while the academically established mean reversion for volatility suggests that periods of a tightly bound price range have a finite duration.
Reflecting these ideas, Athena remains directionally agnostic, selectively overtrading but avoiding short-term noise, to maintain alignment with the market in order to eventually capture a move sufficiently large to recover accumulated losses and yield net profits.
The power of this approach lies in the statistical inevitability of such a major move.
The price to pay is that of patience and of frequent short-term loss realisation —
a difficult challenge for a human trader, but not for a nimble and unrelenting robot!
Athena also presents a valuable advantage for risk management: the accumulation of short term losses is slow and gradual, ensuring an inviolable risk framework and allowing for comfortable monitoring and assessment, while also offering significant immunity to violent market shocks, which are likely to enhance rather than threaten performance.
In offering high certainty in its long term return expectations, Athena is faced with a particular, relatively uncommon, kind of risk: that of a moderate (above noise levels, but not high enough) and protracted sideways volatility. The statistical quantification of the duration and depth of resulting drawdowns is presented in the Risk Analysis section.
The algorithm determines a key level around which it trades, subject to an internally determined noise threshold.
When the FX Price fully traverses the noise channel, the algorithm fully reverses the trade's direction. Each such reversal realises losses, whose magnitude depends on the noise threshold and on transaction costs (bid/ask spread and slippage).
Unless such a reversal occurs, the particular trading position is maintained until an internally determined take-profit level is met. The take-profit level ensures recovery of the cumulative losses of that trading cycle, as well as a variable amount of net profit.
The strategy benefits from diversification across numerous such channels being placed at different key levels, as well as from the natural diversification across the different currency pairs traded. Furthermore, two separate sets of inputs into the major determinants of the algorithm are deployed in simultaneous implementations for each currency pair, to avoid overfitting bias.
Jan | Feb | Mar | Apr | May | Jun | Jul | Aug | Sep | Oct | Nov | Dec | Total | |
---|---|---|---|---|---|---|---|---|---|---|---|---|---|
2020 | -0.2% | 0.5% | 1.5% | 0.3% | -0.9% | -0.6% | -2.8% | 5.1% | 2.9% | ||||
2021 | -0.5% | 0.9% | 1.0% | -1.6% | -5.7% | 6.7% | 1.2% | 0.4% | 1.6% | 0.5% | 0.5% | -0.6 | 4.4% |
2022 | -0.8% | -2.8% | 5.7% | 1.9% | -0.2% | 0.2% | 1.3% | 0.0% | 2.7% | -0.2% | 1.2% | 0.6% | 9.6% |
2023 | 0.6% | -0.6% | -1.2% | 1.6% | -0.3% | -2.5% | -2.6% | 7.6% | 5.0% | 0.7% | 0.4% | 1.0% | 9.7% |
Statistics | Backtest (208 months) |
Live (43 months) |
---|---|---|
Sharpe Ratio | 0.46 | 0.84 |
Avg Monthly Return (annualised) | 9.3% p.a. | 7.2% p.a. |
Monthly Volatility (annualised) | 20.1% | 8.6% |
Avg Number of Trades (per month) | 215 | 187 |
Percentage of winning trades | 31.1% | 42.9% |
Maximum Monthly Loss | 15.6% | 5.7% |
Average Drawdown (including intra-week) |
8.7% | 3.5% |
Maximum Drawdown (including intra-week) |
31.4% | 13.2% |
The Live Performance reflects the auditable track record of AutoMetis in its live trading account with IG Markets.
The nature of the strategy implies that the expected return will be delivered repeatedly with high certainty. The risk analysis focuses on the depth and duration of the dips between such target-clearance points; each a "drawdown episode" if larger than 1%.
The primary relevant risk metric is the maximum drawdown — with sufficient capital to cover the maximum drawdown, the strategy will confidently yield the expected result.
The second relevant risk metric is the duration of underwater periods — the time commitment required for the strategy to recover accumulated losses.
Distribution of Historical Drawdowns
From a risk perspective, we are interested in deriving a high-confidence estimate of the maximum drawdown possible and the maximum duration possible.
It is reassuring that the strategy's largest drawdown as history actually unfolded would only have been 31%, and the longest underwater period was 42 weeks — however, historical frequency is not sufficient to robustly derive a high-confidence estimate.
We improve upon the historical analysis by stress-testing the strategy with a rigorous Monte-Carlo simulation. Our methodology involves generating fictitious timeseries of price evolution by partitioning, randomly mixing, and re-joining the price data, and repeatedly backtesting the strategy on such simulated data.
In doing so, we increase the randomness of the underlying data, which would have a harsh impact on any systematic strategy. We moderate this effect by keeping the unit blocks large enough to still preserve medium-term behavioural effects.
Distribution of Stress Test Simulations
Key Takeaways from Monte Carlo Stress Test
- The median maximum drawdown in a 3-year horizon is 20%.
- The average (expected) maximum drawdown in a 3-year horizon is 36%.
- With 90% certainty, the maximum drawdown in a 3-year horizon will not exceed 55%.
- With 97% certainty, the maximum drawdown in a 3-year horizon will not exceed 100%.
The residual risk tail of 3% represents the probability of a total loss, in the absence of any overarching risk framework. While this is not a negligible percentage, it is the irreducible consequence of forcing randomness upon the data in the Monte Carlo simulation — any systematic strategy tested on a repeated stretch of its worst-performing interval will burn through any finite amount of capital.
Incorporating the stress scenario into our long term return expectations, a repetition of 100 x 3-year cycles would be expected to yield 9% p.a. for 97 of those cycles, and a total loss of -100% for 3 of those cycles.
The overall expected return would therefore be 7.7% p.a.
- The median maximum underwater period is 22 weeks.
- The average (expected) maximum underwater period is 24 weeks.
- All Monte Carlo runs show a maximum underwater period of under 80 weeks.
While the maximum underwater period is a good metric to assess the time dimension of risk, it does not fully convey the expected time horizon required by the strategy to achieve its target return, since it excludes time spent in positive but below-target territory, or shorter dips interrupted by positive performance.
The expected duration for the strategy to reach its target return is 1.8 years, and below 3 years at the 95% confidence interval.
-
Could the strategy be applied to asset classes other than FX?
Perhaps. As discussed in the Concept section, the FX market evidences the smallest adherence to mean reverting behaviour and is therefore the domain of choice for this strategy.
Additionally, some features of the FX market make it comparatively attractive: deep liquidity which allows for scalability and small execution slippage; 24/5 trading continuity which minimises gap risk; lower spreads and lower overnight financing costs than most other markets.
Consequently, we have chosen to limit our research and strategy implementation to the FX market for now.
-
Could the strategy be run over additional currency pairs?
Yes, although we do not expect to be able to extract sufficient diversification benefits in order to significantly improve the overall risk-adjusted performance.
While the strategy is not high frequency, transaction costs are a significant hurdle to clear for any systematic strategy. The particular currency pairs are among the most liquid and cheap to trade, while at the same time each of these USD crosses has a macroeconomic link that supports the expectation of a sufficiently high long term volatility.
-
What is the minimum capital required to run this strategy?
For the risk/return profile presented above (i.e. 9% p.a. return / 20% volatility / 36% expected max drawdown) the required risk capital base is $250,000.
The strategy can be run on the basis of less risk capital, but the risk/return profile will be negatively affected — this is due to the fixed minimum size of individual trades imposed by the broker.
-
Is the strategy fully scalable upwards?
Yes. Our execution algorithm as it stands could support a 100-fold increase to a $25m capital base with no additional changes.
Beyond that, it would probably be wise to overlay an order submission mechanism to spread out the trading. The strategy is not high frequency and therefore has room for additional timing optimisation, which is not currently relied upon.
-
Does the strategy require leverage? What are the risk and capital implications?
Trading in FX is typically done using some kind of a derivative instrument, such as a Forex contract, a CFD, or a Spread Bet. These instruments are all traded on margin, meaning that the deposit capital required by the broker is a fraction of the net notional value of the trading position — or equivalently, the broker funds a trading position which is a multiple of the deposit capital required, implying leverage. For major FX pairs, depending on the client categorisation by the broker, the required margins are relatively small; a professional client could trade at 0.3% (over 300x leverage).
The risk consequences are not inherent to the leverage available but to the Value at Risk at any time, which depends on the notional exposure in combination with the allowed loss.
Indicatively, during our Live Period, our peak aggregate notional exposure reached just 6x Risk Capital, while the largest loss on a single trade was -0.83%. This mild leverage implies less than 2% of our Risk Capital being locked up for margin requirements — a small enough amount to be negligible for the sake of simplicity in the presentation of the strategy.
-
How is the strategy different from a long options straddle strategy?
Access, liquidity, cost, and horizon:
The strategy trades in the spot FX market, so is accessible by virtually anyone, it does not depend on a particular market maker, and it is not exposed to liquidity dry-up risk.
The transaction costs are fixed and small, compared to buying two (likely overpriced) at-the-money options.
Finally, the strategy achieves a similar payout profile but without the constraint of a finite horizon for the realisation of its target.
-
Does the strategy's success imply that the FX market is inefficient?
No. The performance does not necessarily represent excess or abnormal return, nor is there any evidence that the market does not properly and quickly discount all available information.
The return achievable can be viewed as market compensation for the strategy's variance risk, as well as for the strategy's negative skewness.
-
Are you planning improvements to the strategy in the near future?
We believe the strategy currently reflects the underlying concept well, and we have already made the necessary improvements to our execution algorithm.
We are reluctant to tweak the inner working of the strategy, as it would have questionable incremental value and give rise to potential overfitting biases.
Instead, we are now focused on developing a framework for the management of the strategy as a whole: predicting when the strategy tends to perform well and when not, and systematising a weight allocation to it.
-
Aren't you worried you are disclosing too much detail about the strategy?
On the contrary, we prefer to adopt a transparent approach in order to build confidence and invite further discussion and contributions.
The strategy is not purporting to rely upon some holy grail discovery or secret sauce; the fundamental concepts have long been explored in academia. Instead, our key value is our work on the execution of the idea and the construction of the implementing framework.
We specifically aim to differentiate our approach from the all-too-common "black box" products for sale we see in the market, which as a rule have dubious underpinnings and rely on cosmetic manipulations to paint an unrealistic image of their potential.
-
On what platforms can the strategy be run?
We have been running the strategy on the MetaTrader 4 platform of our broker, IG Markets, using a partner VPS service.
The strategy, currently coded in MT4's MQL language, could be re-coded to run on other platforms or link directly to a broker's API — all that's needed is a live and continuous feed for the underlying prices and a modest amount of historical data; everything else is built in, there is no reliance on external libraries, indicators, or databases.
-
Could I send you some capital to run this strategy for me?
No, sorry — AutoMetis is purely a software development company, and is not licensed to undertake regulated financial activities.
We are exploring partnership options with relevant institutional entities, as well as with platforms offering copy trading capabilities.
If you have your own trading account and would like assistance to code a systematic strategy, we could be able to help — please use the form below to contact us for further discussion.
Raphael R. Maiopoulos
Co-Founder
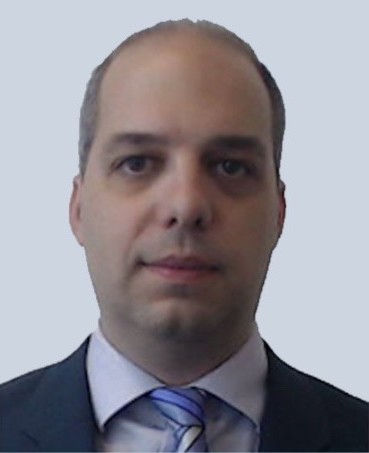
Raphael is a Finance Professional with extensive quant experience in financial and risk modeling, pricing and structuring.
He has worked in Structured Equity Finance & Trading at BofA Merrill Lynch, in Structured Capital Markets at Barclays Capital, and earlier in Aircraft Finance & Leasing.
Raphael holds an MBA from London Business School and a BA degree in Applied Mathematics from Harvard University.
Dr Vasileios Symeonidis
Co-Founder
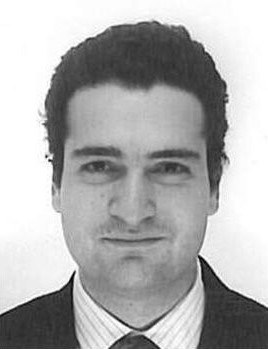
Vasileios is an Applied Mathematician with diverse teaching experience, including at the American College of Greece and Athens College.
He has previously worked in an AIM-listed real estate fund and has served as head of the property division of a large institution.
Vasileios holds a PhD in Computational Fluid Dynamics from Brown University and a Masters in Mathematics degree from the University of Oxford, and was a PostDoc at MIT and Brown; he has published several papers on numerical methods and simulations in the physical sciences.
Dr Filippos Papakonstantinou
Academic Advisor
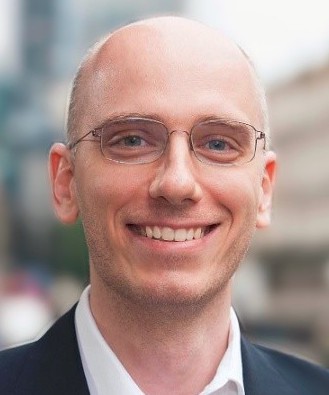
Filippos is Assistant Professor of Finance at King's College London, and previously at Imperial College London Business School.
Filippos holds a Master's and a PhD in Economics from Princeton University and a Bachelor's degree in Economics from Harvard University; he has published papers on behavioural economics and finance, asset management, and Bayesian econometrics, and has taught graduate courses in asset pricing, quantitative methods in finance, and stochastic calculus.
AutoMetis is a software development company, whose primary business is the creation and development of automated trading strategies and the testing of such strategies in real market conditions.
Nothing presented herein constitutes an offer to sell or a solicitation of any offer to buy any financial securities or services. AutoMetis gives no investment advice and makes no representation as to the future performance of the trading strategies presented.
team autometis.com
AutoMetis Ltd
St. Julians Accountancy
Wied Ghomor Street
St. Julians STJ 2043
Malta
Registration No: C 58724
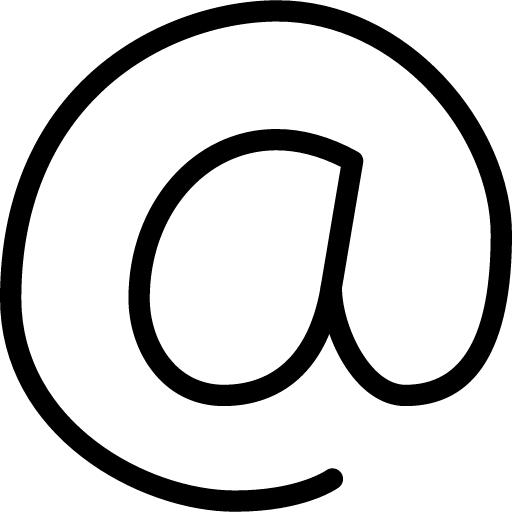